AI Action Summit: AI in the service of research to solve the unsolvable
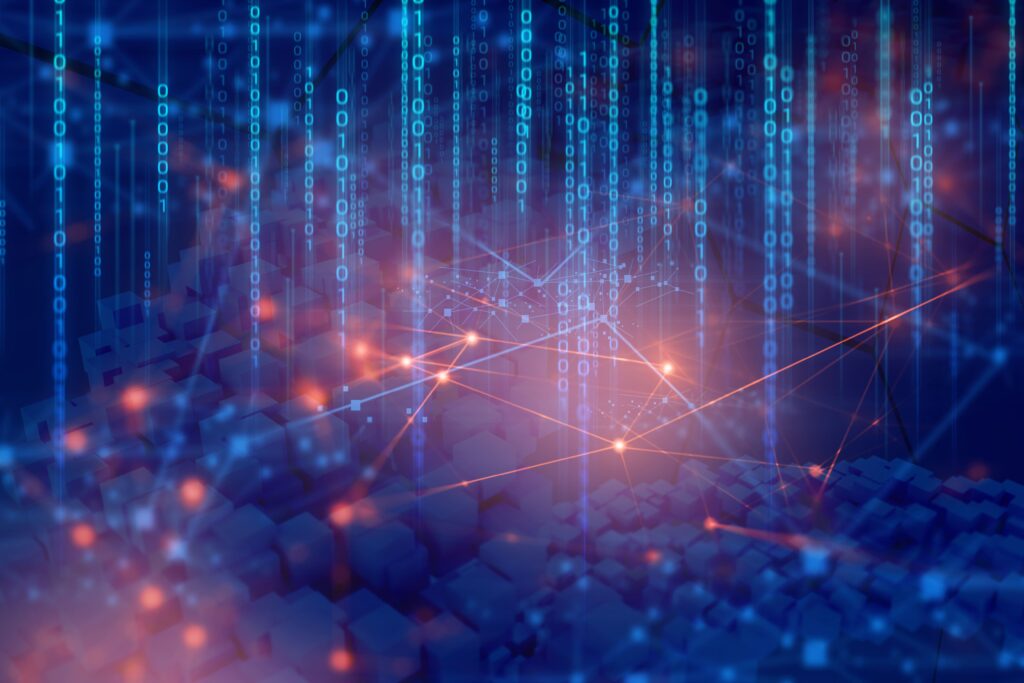
The inverse problem is a fundamental concept in science and engineering: instead of predicting effects from known causes, it consists of tracing observed effects back to the underlying causes. This approach is particularly valuable in contexts where the causes cannot be measured directly and where conventional physical models show their limitations. AI, in particular machine learning (ML), plays a central role here by refining models and exploring otherwise inaccessible solution spaces.
This synergy between AI and inverse problem is very present in the research projects carried out by Mines Paris – PSL, this approach being found in concrete applications of very diverse disciplines, presented here through three examples, from the millimeter scale to the kilometer scale.
In oncology, precision medicine aims to offer each patient a treatment tailored to the specific characteristics of their tumor. This strategy is therefore based on our ability to predict the progression of a disease and the effectiveness of a treatment by integrating heterogeneous data such as genomic sequencing, medical imaging and gene expression.
Here, CBIO uses AI to solve a fundamentally inverse problem: based on the results of the treatments administered and the biological profiles of the patients, the aim is to determine which factors predict the response to the treatment. This work is therefore not limited to proposing a black box for making predictions, but aims to reveal underlying biological mechanisms that are still unknown.
For example, in the prediction of a defect in the natural DNA repair mechanism called homologous recombination (HRD) – a key factor in the effectiveness of certain anti-cancer treatments, such as PARP inhibitors – CBIO researchers, in collaboration with teams from the Institut Curie, have demonstrated that AI models applied to the analysis of tumor section images, or “pathological imaging”, can identify visual clues associated with this defect. These advances open up new prospects for faster diagnoses and more targeted treatments. Additional research projects, conducted in collaboration with the “Women’s Cancers” IHU of the Institut Curie and the Nantes University Hospital, are underway.
Figure – Morphological patterns underlying homologous recombination deficiency, identified by Artificial Intelligence
In the field of solar energy, the resilience of installations in extreme weather conditions is a major challenge. Traditionally, solar panels are equipped with tracking systems that adjust their orientation according to the position of the sun. However, in strong winds, these systems adopt a predefined safety posture that does not guarantee optimal protection.
Elie Hachem and Cemef de Mines Paris – PSL have developed an innovative solution combining the numerical simulation of air and liquid movements, called “computational fluid dynamics” (CFD), and reinforcement learning, an AI technique that learns to make the best decisions through trial and error. The aim is to optimize the orientation of the panels in real time in response to gusts of wind.
By integrating the Navier-Stokes equations, fundamental mathematical equations that describe precisely how fluids such as air flow and exert forces, with learning algorithms, solar panels become autonomous entities capable of anticipating the impact of the wind and adjusting their inclination accordingly. This coupling of AI and physical modeling perfectly illustrates the approach to the inverse problem: by analyzing the effects of the wind on the panels, the algorithm gradually learns the optimal configurations that guarantee both their protection and maximum energy yield.
A digital replica of the solar power plant called a “digital twin”, designed as a virtual copy of the physical installation, has also been developed to test these strategies before they are implemented in real life. This computer model, connected to sensors, makes it possible to simulate the performance of the power plant in real time and to adjust the control parameters in advance.
AI that proposes different orientations of the panels in response to gusts of wind.
In the context of energy transition and geological risk management, it is essential to characterize the subsoil accurately, whether to monitor the evolution of underground reserves or anticipate natural phenomena such as landslides.
Seismic prospecting methods are based on the emission of waves which, as they propagate through the ground, interact with its different layers before being partially captured at the surface. One of the major challenges of this approach lies in the interpretation of the recorded signals: how can we trace the internal structure of the subsoil from these indirect observations?
Hervé Chauris, Nicolas Desassis, lecturer-researcher, and Yuke Xie, doctoral student, all three at the Centre de Géosciences de Mines Paris – PSL, use neural networks to refine these models and estimate the associated uncertainties. The probabilistic approach adopted makes it possible to generate not a single image of the subsurface, but a set of plausible scenarios integrating physical and geological constraints. This methodology guarantees more reliable and more precise modeling, which is essential for applications such as CO2 storage or the exploitation of geothermal resources.
Fig. 1 – Application on synthetic data. (a) Exact velocity field, (b) initial average on the prior fields, (c) average on the posterior and (d) standard deviations (after Xie et al., 2024).
AI goes far beyond the framework of an industrial and technological revolution. It profoundly transforms our relationship to knowledge, work, information, culture and even language. Its development, with transnational implications, calls for an in-depth international dialogue, mobilizing governments, researchers, companies and citizens, in order to develop collaborative solutions and standards for AI at the service of tomorrow’s society.
AI applied to scientific research is not limited to a tool for accelerating calculations: it makes it possible to tackle problems of unprecedented complexity and produce previously inaccessible results. By combining AI and inverse problems, Mines Paris – PSL is paving the way for a new generation of scientific and technological discoveries, where a detailed understanding of the underlying mechanisms becomes a driver of innovation.
In the future, these approaches could be extended to other disciplines, from materials physics to biology, thus consolidating the place of AI as a lever for transformation in scientific research.
Michel, A. Ansaldi, J. Viquerat, P. Meliga, E. Hachem. Combining machine learning and computational fluid dynamics for solar panel tilt angle optimization in extreme winds. Physics of Fluids, 2024, 36 (12), ⟨10.1063/5.0233709⟩. ⟨hal-04844403⟩ https://cnrs.hal.science/ENSMP_CEMEF/hal-04844403v1
Tristan Lazard, Guillaume Bataillon, Peter Naylor, Tatiana Popova, François-Clément Bidard, et al.. Deep learning identifies morphological patterns of homologous recombination deficiency in luminal breast cancers from whole slide images. Cell Reports Medicine, 2022, 3 (12), pp.100872. ⟨10.1016/j.xcrm.2022.100872⟩. ⟨hal-03936608⟩
https://minesparis-psl.hal.science/ENSMP_CBIO/hal-03936608v1
Xie, Chauris, H. and Desassis, N., 2024, Stochastic full waveform inversion with deep generative priori for uncertainty quantification, https://arxiv.org/abs/2406.04859.
As part of the Summit for Action on AI 2025, on February 8 Mines Paris – PSL is hosting the Tournées de l’Excellence Génération IA, an event dedicated...