Our doctoral student Akylas Stratigakos wins the 1st Think SmartGrids 2023 thesis prize
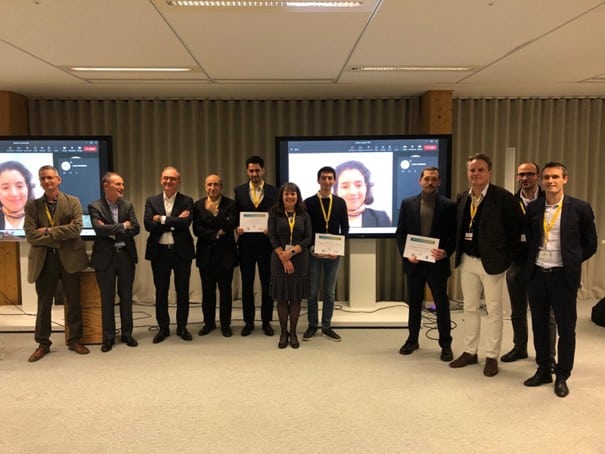
The male prize was awarded to Akylas Stratigakos for his thesis entitled “Towards the Paradigm of Prescriptive Analysis for Energy Forecasting and Power System Optimization”.
Akylas defended his thesis in July 2023 under the supervision of Georges Kariniotakis, Head of Renewable Energies & Smartgrids Group and Andrea Michiorri, Associate Professor at the PERSEE Center of Mines Paris – PSL. The awards were presented in Paris at the Association’s Vœux 2024 ceremony.
Through this award, Think SmartGrids underlines the importance of network digitization, and recognizes the work of the ERSEI group in this field. The group’s research is aimed at facilitating the growing integration of renewable energies into the electricity mix, and simplifying network operation through optimized arbitration based on machine learning and operational research tools.
Congratulations to Akylas Stratigakos for his work!
To mitigate the adverse effects of climate change, the power sector is rapidly moving towards decarbonization through the integration of renewable energy sources, such as wind and solar. In this context, advanced data-driven methods, leveraging the tools of machine learning and operations research, hold great promise as key enablers to address the uncertainty and variability of weather-dependent renewable energy sources. In this thesis, we take a holistic approach by examining the chain of models from data to uncertainty modeling to decisions, and develop data-driven methods that enable improved and resilient decision-making in modern power systems. To maximize the value of forecasts, we develop a method that integrates forecasting and optimization, and propose a framework for assessing the impact of data on decisions. To foster the adoption of advanced data-driven methods and accelerate traditional workflows, we develop an interpretable method for predicting solutions to constrained optimization problems. To strengthen the resilience of models in the face of problematic data, we propose an approach that enables missing data to be handled within an operational framework. We also propose an optimization-based method for clustering data across a number of independent problems, thereby improving overall performance and decision robustness. The proposed methods are validated in various experiments related to power system operation and participation in electricity markets.
Félicitations à Corentin Gombert, lauréat du prix de l’ARIMHE 2023 pour sa thèse « Incarner la démocratie dans l’entreprise : une recherche-interventi...