HAIBrid: hybrid AI for automated driving presented at Mines Paris Research Day
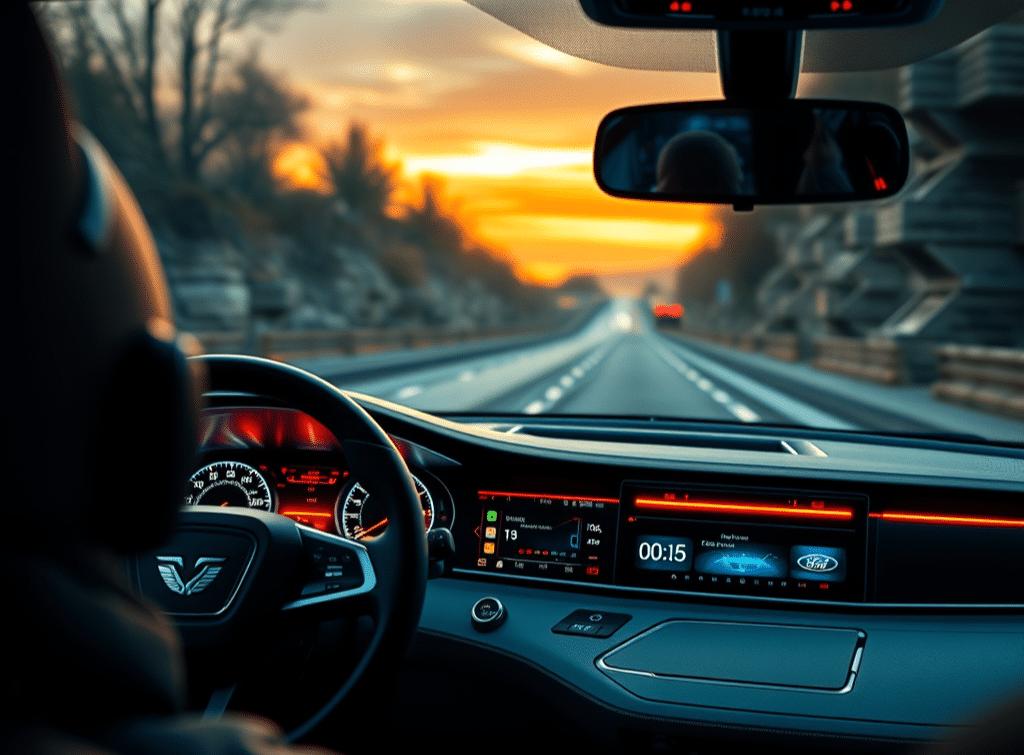
The development of autonomous vehicles is experiencing unprecedented growth.However, to achieve optimum safety and performance, these systems must be able to understand and anticipate the behavior of other road users.
Mines Paris Research Day, held in Paris on July 2, 2024 at Mines Paris – PSL, offers an opportunity to discover the cutting-edge research being carried out at the School, facilitating exchanges between researchers, major companies, and start-ups. This event highlights the synergy between science and industry, through five key challenges: the energy transition, responsible resource management, cultural and creative industries, data science and AI, but also the mobility of the future. It is with this in mind that the HAIBrid cooperative project, part of a Franco-German collaboration, proposes to integrate hybrid AI techniques to model road behavior and improve automated driving systems.
The key to automated driving lies in a precise understanding of the interactions between different road users. However, collecting large-scale data, such as vehicle and pedestrian trajectories, presents significant challenges. Indeed, manual annotation of such data is tedious, and the great variability of situations makes it difficult to classify interactions.
HAIBrid aims to overcome these obstacles by drawing on recent advances in unsupervised learning, a branch of machine learning characterized by the analysis and clustering of unlabeled (nameless) data, whose algorithms learn to find patterns or groups in the data, with very little human intervention. This approach would automatically extract trajectories and classify interactions from traffic camera, drone and on-board sensor data. Using this data, the project also aims to generate 3D maps enriched with semantic information crucial to the planning of automated vehicles.
One of HAIBrid’s main challenges lies in the automatic extraction and labeling of large-scale trajectories. Current object detection and tracking methods, while effective for certain tasks, still encounter difficulties when it comes to dealing with complex scenes, notably due to occlusions and the diversity of objects to be tracked (vehicles, pedestrians, etc.).
The project therefore proposes a hybrid approach, combining physics-based models and unsupervised deep learning techniques. This hybridization will enable better processing of video data and the construction of accurate trajectories, while taking into account the particularities of the 3D environment.
In addition to trajectory extraction, HAIBrid focuses on the creation of automatic 3D maps. These maps provide an essential framework for understanding road interactions, integrating information such as road markings, traffic signs and intersection configurations.
However, creating these 3D maps is a complex and costly process, usually carried out using sophisticated survey vehicles. HAIBrid proposes to reduce reliance on such expensive equipment by leveraging computer vision and deep learning techniques, offering a more affordable solution for large-scale mapping.
Another key innovation of the project lies in the modeling of behaviors and interactions between road users. Rather than simply predicting individual trajectories, HAIBrid focuses on collective interactions. The aim is to create predictive models capable of anticipating not only the actions of automated vehicles, but also those of other road users, be they cars, pedestrians or cyclists.
To achieve this, the project relies on techniques to identify recurring behaviors in traffic data. These models can then be used to improve the decision-making of autonomous vehicles and make their driving more fluid and natural, by adapting to the behavior of other road users.
The HAIBrid project is not confined to producing theoretical innovations. In addition to new datasets collected by cameras, drones and sensors, the project will develop methods and tools for capturing and labeling trajectories and interactions. These advances will benefit the automotive industry by providing more efficient solutions for automated driving.
Improved algorithms for predicting collective interactions, combined with detailed semantic map information, will enable more accurate decision-making within autonomous vehicles. Ultimately, HAIBrid will help to make automated vehicles safer, more cooperative and better able to adapt to the complexities of road traffic.
The ” Ecodesign in reality “educational project , developed by the Institut supérieur d’ingénierie et de gestion de l’environnement (ISIGE), the FabL...