AI and geostatistics for climate risk: 3 questions to Thomas Romary and Denis Allard on the 2nd anniversary of the Geolearning Chair
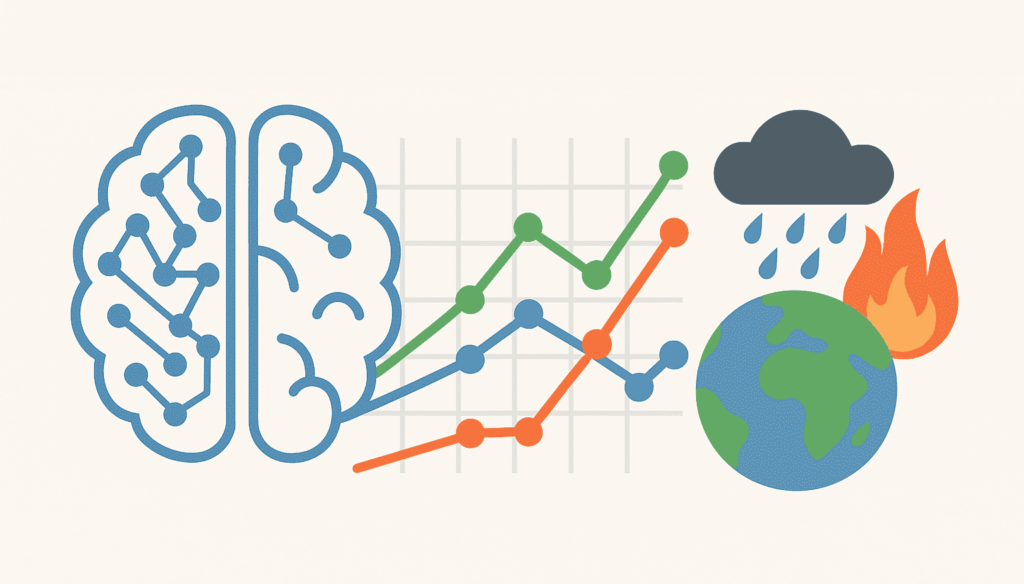
Launched in 2023, the Geolearning Chair, supported by the Centre de Géosciences de Mines Paris – PSL in partnership with the Biostatistique et Processus Spatiaux (BioSP) unit of the Institut national de recherche pour l’agriculture, l’alimentation et l’environnement (INRAE), develops advanced analysis tools to better model and analyze natural phenomena such as extreme weather events or environmental monitoring. The Chair uses an interdisciplinary approach combining geostatistics, machine learning and extreme event modeling to better quantify the frequency and magnitude of these risks. Financed by the Agence nationale pour la gestion des déchets radioactifs (ANDRA), BNP-Paribas, the Caisse centrale de réassurance (CCR) and the Fondation d’entreprise SCOR pour la Science, it provides decision-makers with methodologies that draw on the most recent theoretical advances in these three fields. Two years after its inauguration, we take stock with Thomas Romary, Professor at Mines Paris – PSL, and Denis Allard, Director of Research at INRAE, of the progress made and the outlook for 2025.