What is generative in generative artificial intelligence? Mines Paris Research Day sheds light on ChatGPT’s generative logic
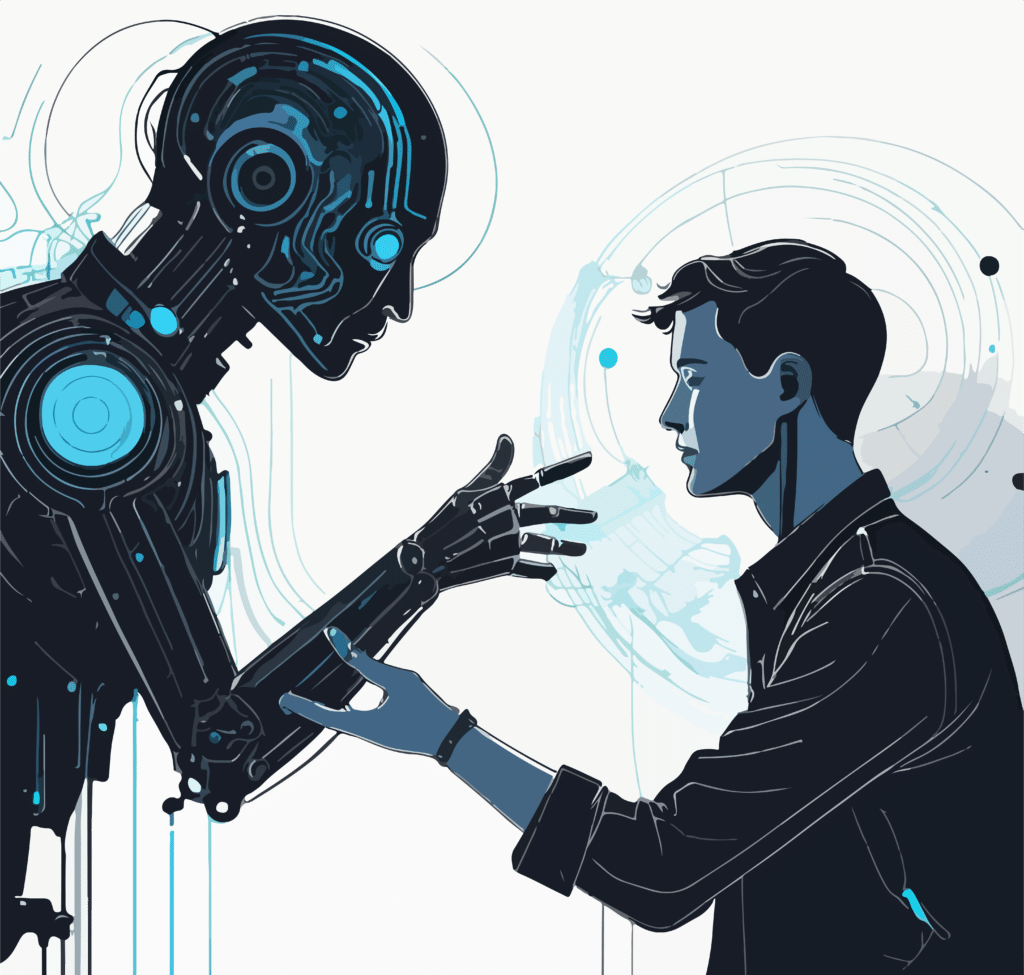
Generative artificial intelligence models have turned our approach to digital creation and innovation on its head. Since the advent of ChatGPT, these models have captured the world’s attention, promising unprecedented opportunities while posing complex challenges. However, one question remains: what do these tools actually generate?
Mines Paris Research Day, held in Paris on July 2, 2024 at Mines Paris – PSL, offered the opportunity to discover cutting-edge research conducted at the School, facilitating exchanges between researchers, major companies, and start-ups. This event highlights the synergy between science and industry, through five key challenges: energy transition, responsible resource management, cultural and creative industries, mobility of the future, but also data science and AI. During this event, CGS researchers mobilized advances in generativity theories (design theory) to shed light on what is generative in generative AI.
Mines Paris Research Day July 2 in Paris
Prompt used on generative AI dream.ai: a human chatting with a generative artificial intelligence
GenAI is described as follows by Ian Goodfellow, a pioneer in the field:
GenAI is able to go beyond what it has seen before and create something new.
Unlike traditional AI, which seeks to understand and reproduce human intelligence, GenAI focuses on the creation of original content, be it text, images or video. Since the emergence of ChatGPT, these GenAI models have raised as many hopes as fears, and their ability to produce original content is at the heart of the debate.
But what does “original” really mean? This is where the notion of generativity becomes crucial. Generativity refers to a system’s ability to produce or create something new. But novelty can be subjective and difficult to define. The researchers therefore turned their attention to the various mechanisms at work in GenAI models to better understand this generativity.
Since the 1970s and 1980s, design theories have focused on the logics of generativity, and recent advances have made it possible to account for a variety of generativity models: generativity by activation, generativity by inversion, fixed-language generativity and generativity with language expansion.
The arrival of OpenAI’s ChatGPT marked a turning point in the adoption and discussion of GenAI. Despite the abundance of research on GenAI, the generativity of these models has not been studied in detail. It is therefore essential to characterize the generativity of GenAI models.
Based on design theories, the researchers reviewed several families of GenAI models. They then characterized the generativity of each family, identifying new and sometimes surprising forms of content creation. Understanding the generativity of GenAI models is crucial: it enables us to better understand the creative potential of these algorithms, and provides clues as to how to use each model according to specific creative needs.
Boat – Bill Hertha, prompt used on Midjourney: Paul Klee, sea, mezzotint, abstract, steamboat
From a mathematical point of view, GenAI uses models to learn the underlying structure of a data set and generate new data points similar to those in the original set. These models, like the famous neural networks, go through a training phase where their structure and parameters are fixed. Once trained, these models can generate original content, be it text, images or even video.
The researchers focused on four families of generative AI models:
This family is a type of neural network architecture designed to efficiently compress (encode) input data to its essential features, and then reconstruct (decode) the original input from this compressed representation. Most of these types are used for feature extraction such as data compression, image denoising, anomaly detection and facial recognition.
This family is a type that generates new content by mimicking a process similar to the random movement of tiny particles in a liquid. It creates new images by transforming clear images into noise, and then converting this noise back into clear images. Tools such as MidJourney and DALL-E produce high-quality images in this way, and allow fine control over the process, making it possible to improve the resolution of a video game image or fill in missing parts of a photo.
This family is a type of deep learning architecture, involving two neural networks. The two networks, a generator and a discriminator, compete with each other, one to generate new data from a training dataset, the other to classify whether a dataset is generated by the former or not. The system then trains itself competitively until the discriminator network can no longer distinguish false data from original data. In this way, it is possible to generate new images from an existing image database, or original music from a song database.
This family is a type that analyzes natural language queries and predicts the best possible response based on their understanding of the language. To do this, it draws on knowledge gained after being trained with hundreds of billions of parameters on colossal linguistic datasets. It takes context into account and processes different parts of the input, making it capable of generating lengthy responses. Companies in all sectors use it for question-and-answer robots, text summarization and content generation.
The researchers were thus able to provide a generative analysis for each family. This systematic analysis shows that the tools use two types of generativity:
Analysis shows that these families belong neither to the model of generativity by inversion nor to that by language expansion. In other words, they operate differently from the two types of generative AI with which we are already familiar.
Prompt used on Dall-E: an astronaut on horseback in photorealistic style
Recent work on generative artificial intelligence (AI) offers some interesting ideas for better managing these technologies in ways that benefit society. Here are a few avenues to explore:
This work has also revealed several initiatives aimed at reversing, in part, pre-trained patterns. This opens up an exciting new field of research, based on innovative technical tools and algorithms, such as evolutionary algorithms of the Quality Diversity type.
On this basis, the Transitions Bauhaus has launched a collaborative program to explore the best ways to handle generative AI in the future. This program aims to make the most of these technologies while minimizing the associated risks. Research into generative AI enables us to study and optimize the creation of personalized content on a large scale, improving customer engagement and the relevance of communications. Investing in research into generative AI means we can stay at the cutting edge of technological progress, and respond more effectively to the changing needs of businesses.